Composite Artificial Intelligence will completely Change the Business Landscape AI Innovation Direction 2 KellyOnTech
This issue continues to answer the question, what are the directions of AI innovation?
According to the AI technology maturity curve published in 2022 by Gartner, the world’s most authoritative IT research and advisory consulting firm, AI innovations fall into four main categories:
- Data-centric AI
- Model-centric AI
- Application-centric AI
- And Human-centric AI
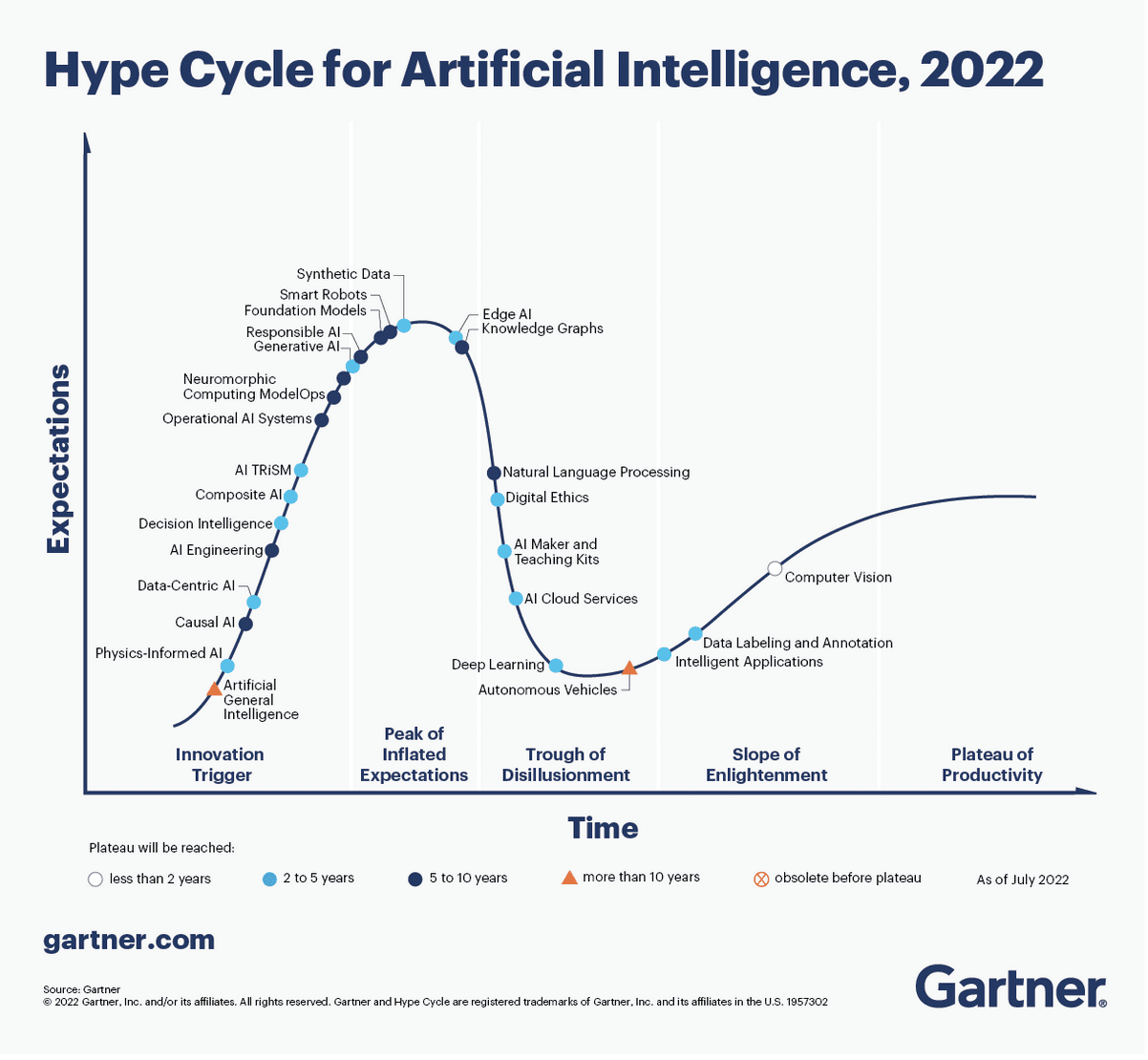
The previous issue introduced data-centric AI startups and use cases. This issue introduces model-centric AI innovation.
Model-centric AI innovations mainly include physics-based AI, composite AI, causal AI, generative AI, basic models, and deep learning. This issue focuses on composite AI.
What Is Composite AI
Composite artificial intelligence refers to the fusion of different artificial intelligence technologies to improve learning efficiency and broaden the level of knowledge representation. Since no single AI technology is a panacea, composite AI ultimately provides a platform to more effectively solve a wider range of business problems.
Gartner expects the business benefits of composite AI to be potentially transformative and to become mainstream within two to five years, supporting all kinds of new ways of doing business across industries that will revolutionize the business landscape. For example, composite AI:
- Can bring the power of AI to a broader group of organizations that do not have access to large amounts of historical or tagged data, but have a wealth of human expertise;
- Helps to expand the scope and quality of AI applications (i.e., more types of reasoning challenges can be embedded).
What Are the Applications of Composite AI
There are many typical examples of well-known model-centric AI solutions, including AlphaGo developed by DeepMind, which was acquired by Google. AlphaGo is an AI system that defeated world Go champion Lee Sedol in 2016. It combines deep learning techniques with reinforcement learning to become proficient at complex board games. This breakthrough result has revolutionized the field of gaming AI, and demonstrates the enormous potential of deep neural networks for strategic decision making.
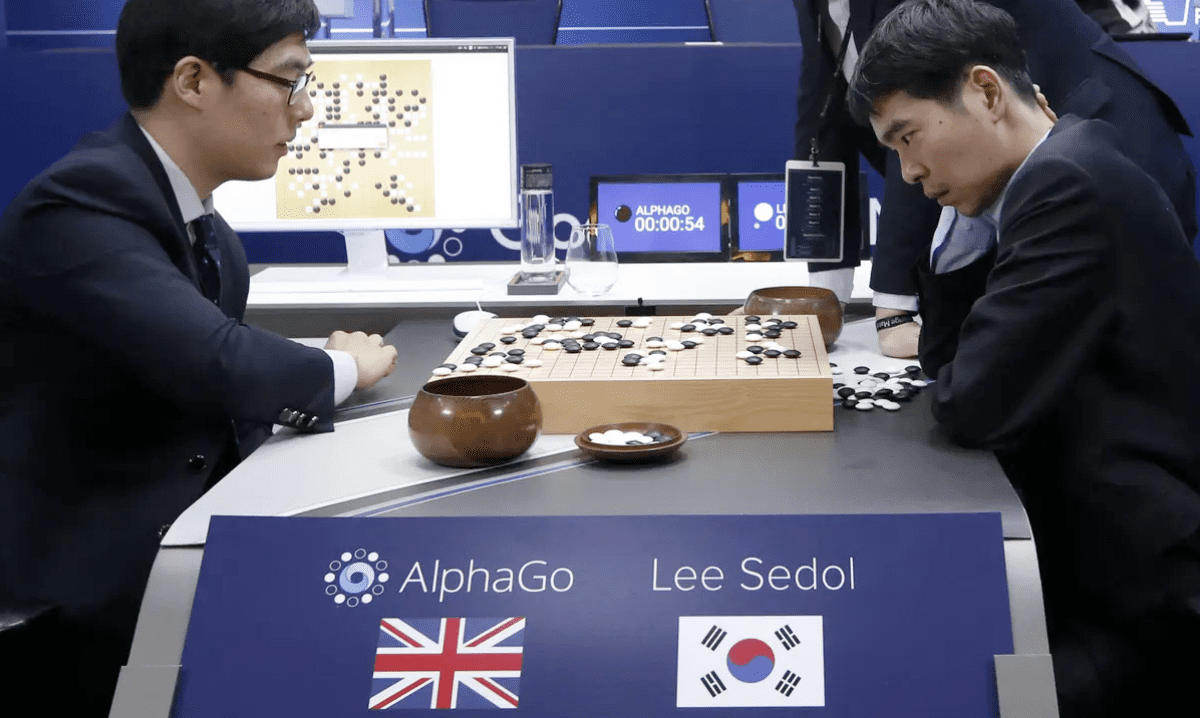
Another well-known model-centric AI solution is Tesla’s Autopilot. This compelling system uses a model-centric AI approach to autonomous driving. It fuses computer vision, deep learning, and sensor data to accurately understand and interpret its surroundings, including recognizing objects, detecting lanes, and making real-time driving decisions. The AI models in Autopilot are constantly learning and improving from the data collected by Tesla vehicles to provide an even better driving experience.
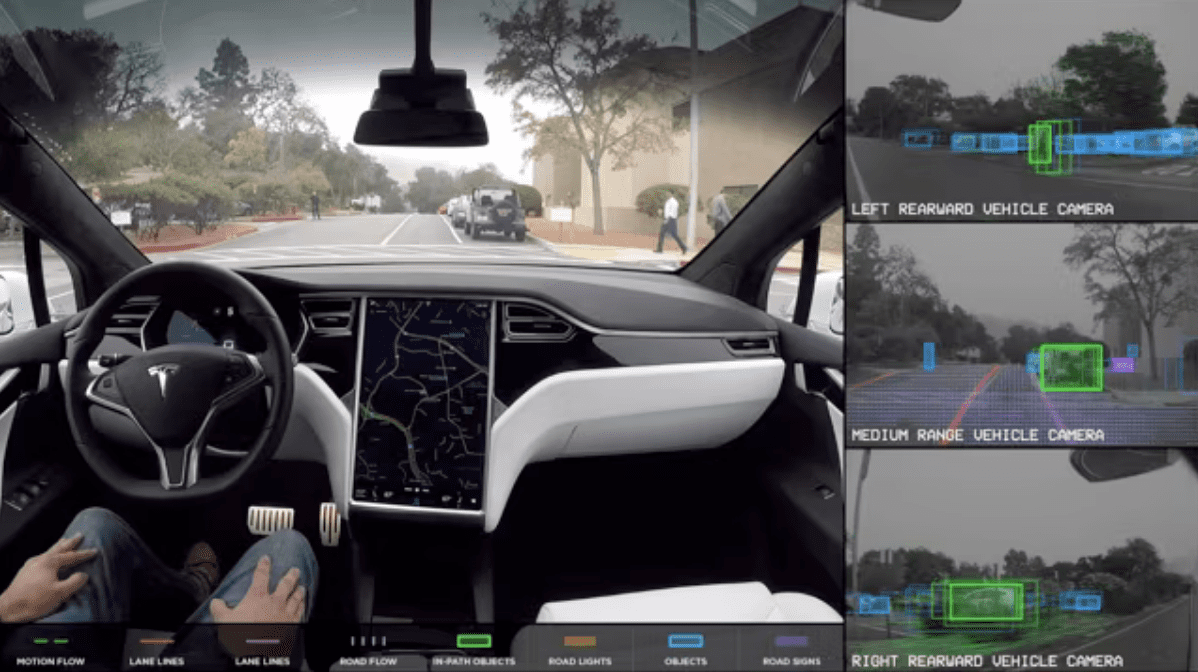
Which Model-Centric AI Solution Provider Is Worth Watching
Here I introduce DataRobot, an early start-up founded in 2012 and headquartered in Boston, Massachusetts. DataRobot’s enterprise AI platform makes data science accessible to the masses through end-to-end automation of building, deploying and managing machine learning models. The company has been committed to providing advanced AI technologies and ROI support services to global enterprises competing in today’s intelligence revolution. It once became the hottest AI unicorn in the United States.
DataRobot positions itself as the “intelligence layer” of the data stack. This is an evolving category between the data layer. ( i.e. Snowflake, DataBricks) and application layer ( i.e. SAP, Salesforce).
Last summer 2022, DataRobot was struggling. The startup unicorn laid off a quarter of its staff and appointed a new CEO, former Google and Amazon executive Debanjan Saha. Why is this? The main reason is that many companies have invested heavily in artificial intelligence, but few have been able to demonstrate real business results. What to do? To this end, DataRobot has upgraded its AI platform.
DataRobot New AI Platform 9.0
In March 2023, DataRobot returned with the launch of its latest AI platform 9.0, along with deeper partner integrations, AI accelerators, and a redesign service offering — all dedicated to helping its clients get measurable value from their AI investment.
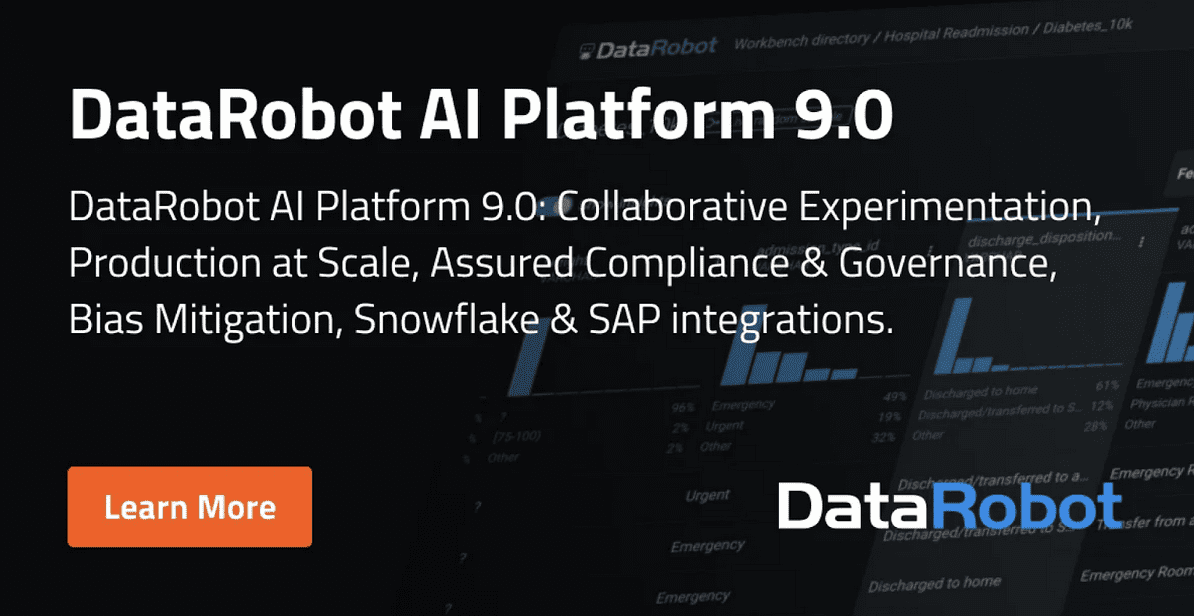
The new AI platform includes Workbench, a user experience that supports users with code-first and no-code approaches; reducing enterprise risk through decreasing bias, centralizing model monitoring and automating model compliance, and a new bundle of AI services.
The cloud-based SaaS version of the DataRobot AI platform is available on Amazon AWS, Google Cloud and Microsoft Azure. For local and private cloud customers, DataRobot supports Red Hat OpenShift to accelerate installation and deployment, and integrate with existing enterprise IT investments.
DataRobot also announced several new and deeper partnerships, including enhanced Snowflake integration for data preparation, model building and monitoring. In addition, it announced a partnership with SAP to help companies leverage business data from SAP HANA Cloud and other third-party data sources, build custom machine learning models in DataRobot, and embed them into SAP application stacks.
Use Case — Supply Chain Management
Over the past few years, the coronavirus (COVID-19) pandemic has brought unprecedented uncertainty to supply chains — making an already difficult task of managing it more difficult. As a result, many manufacturers are struggling to keep production and inventory in line with changing procurement needs. Manufacturers need to prevent parts shortages. Parts shortages lead to underutilization of machines and transportation, leading to a Domino effect of delayed deliveries through the network.
Parts shortages are often caused by shipment delays. In many cases, delayed shipments persist, and supply chain managers need to assess the root causes based on historical data, and then implement short- and long-term adjustments to prevent them from occuring in the future. But due to the time and resources required, supply chain managers cannot effectively analyze the historical data available in the Material Requirements Planning (MRP) system.
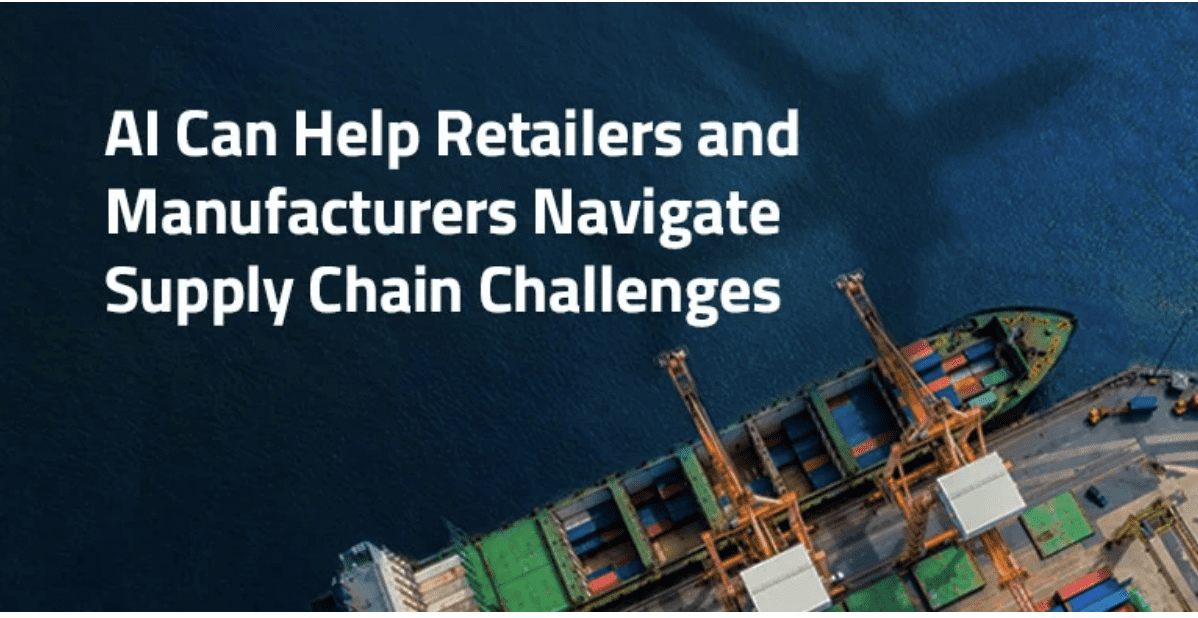
This is where composite AI solutions come in handy in supply chain management. It can greatly enhance manufacturers’ ability to predict future demand for their goods, even in uncertain and dynamic times. Composite AI solutions in supply chain management can:
- Forecasting demand: Using a range of historical data sources to understand future need levels, manufacturers are able to increase availability by more than 5% in many cases, reduce waste by more than 8%, and reduce the losses due to write-offs.
- Predicting returns: By predicting how much inventory will be returned, retailers need to source less inventory from suppliers, thereby maximizing the risk of over overstocking throughout the supply chain.
- Reduce out-of-stocks: With better forecasting, retailers can rely on better granularity to reduce out-of-stocks.
- New product forecasting: Machine learning can predict the likely sales of new product sales in the first few weeks and months.
- Price optimization: A comprehensive analysis of multiple factors of competitors, competing products, brands, categories and locations, .etc., to give the optimal price point, thereby optimizing the balance between demand and supply.