The Next Big Wave of AI Investment: Diving into the Synthetic Data Opportunity KellyOnTech
This issue answers the question, what are the directions of AI investment?
According to the world’s most authoritative IT research and advisory consulting firm Gartner, artificial intelligence innovations are divided into four main categories:
- Data-centric AI;
- Model-centric AI;
- Application-centric AI;
- and human-centric AI.
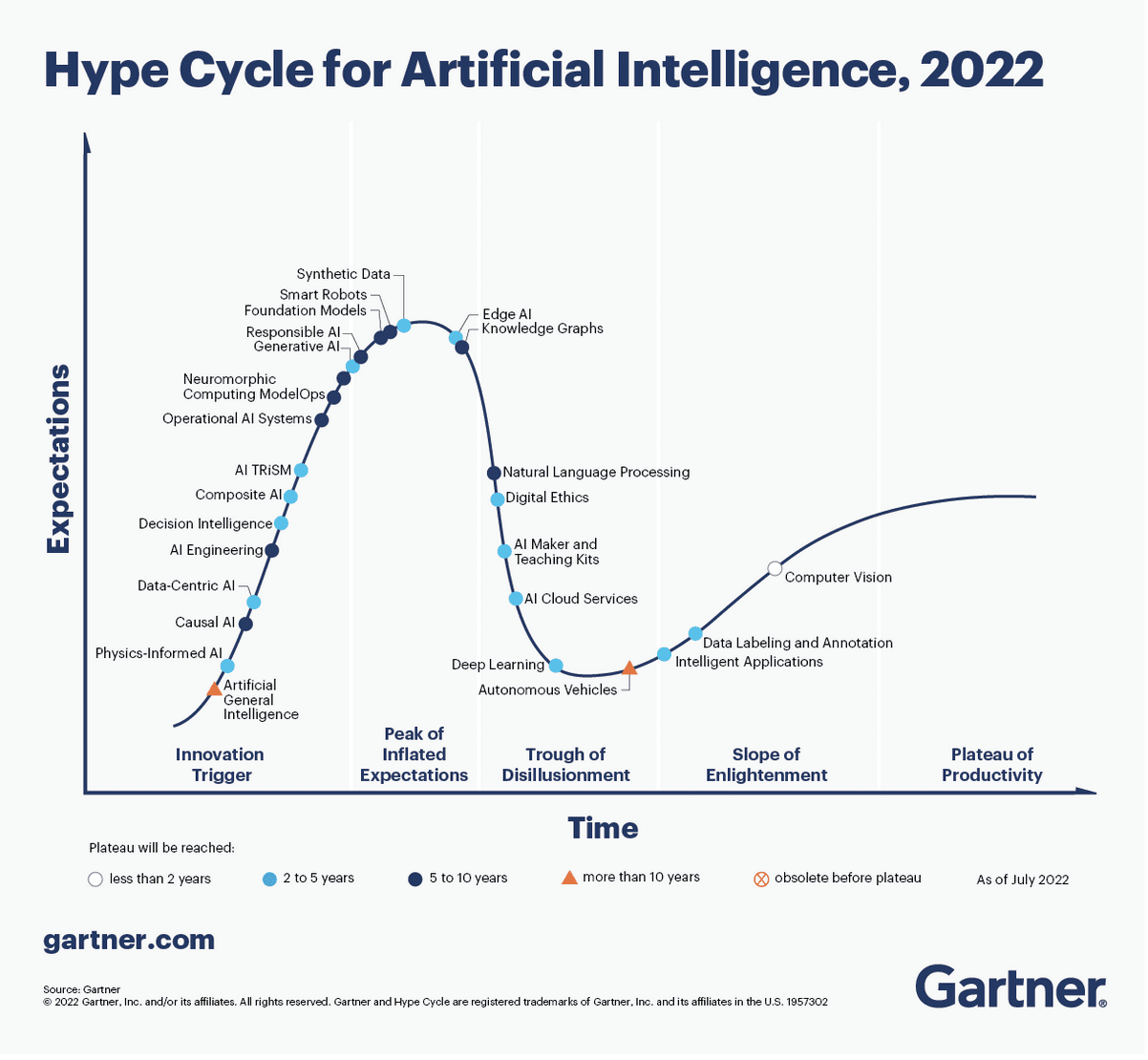
This issue starts with the data-centric AI. Data-centric AI innovations mainly include synthetic data, knowledge graph, data tagging and annotation. Let us focus on synthetic data innovation.
What are the Current Pain Points of Machine Learning and Artificial Intelligence Model Training
If you are a practitioner of machine learning, you have more or less encountered the following problems when training machine models with data:
- Real data is scarce or expensive to obtain
- If the data used contains personally identifiable information or other sensitive information, it is likely that such data cannot be used due to privacy protection
- During the testing phase, developers need data that simulates various scenarios to evaluate the model.
What to do? This is where synthetic data comes into play. The various problems mentioned above can be effectively addressed by using synthetic data.
What Is Synthetic Data
Synthetic data is artificially generated data that mimics the statistical characteristics and patterns of real-world data. It is created using various algorithms and models, rather than being collected from actual observations or measurements.
According to Gartner, by 2024, 60% of the data used to train AI will be synthetic data, and by 2030, synthetic data will completely surpass real data as the primary source of data used to train AI models.
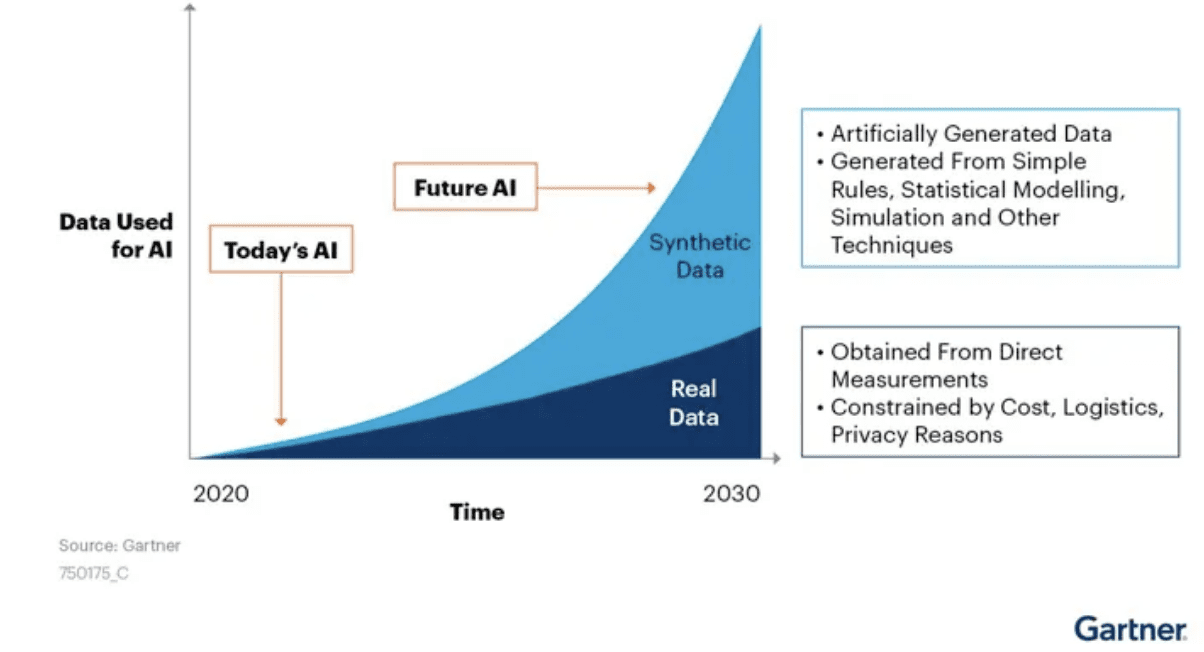
The specific value of synthetic data is mainly reflected in the following aspects.
- First, synthetic data can quickly and accurately construct data sets, which greatly saves data collection costs, and meets large-scale data needs;
- Second, synthetic data effectively solves data privacy and data security issues, and have great application value in fields where user information is sensitive, such as finance and medical care.
- Third, synthetic data ensures data diversity, predicts edge cases, and avoids algorithm discrimination to create a fairer and more inclusive artificial intelligence model. It can also fill in the missing data, insufficient data, and unbalanced data that may exist in real data sets, and improve the robustness and generalization capabilities of machine learning algorithms.
- Fourth, synthetic data improves the flexibility of data utilization and makes data utilization more customized. It can generate data with specific attributes according to specific scenarios and requirements.
In addition, AI can self-learn in a virtual simulation world constructed from synthetic data, which will greatly expand the potential boundaries of AI.
Which Company Is Worth Watching?
There are many companies that focus on synthetic data, depending on the direction of data synthesis, such as synthesizing data for computer vision applications.
The Israeli company Datagen was established relatively early. Founded in 2018, it raised a US$50 million Series B financing in February 2023 this year, just 11 months after its $18.5 million Series A round last year. The company specializes in realistic visual simulations and reconstructions of the natural world, with obvious expertise in human motion.
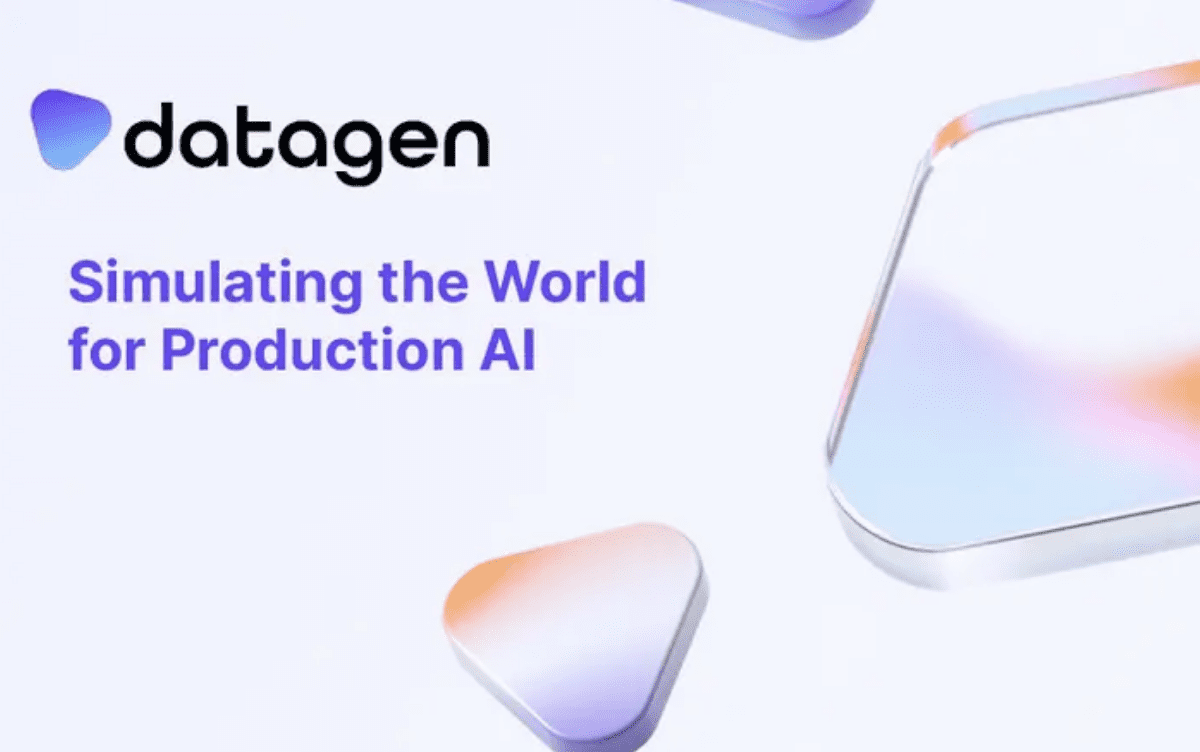
Datagen uses the previously described generative adversarial network to generate synthetic data, which is similar to a computer chess game between two systems, with one system generating virtual data while the other evaluates the accuracy of the results.
Why Datagen’s Valuation Is So High
Data-as-Code Solution
Datagen’s high valuation is due to its development of an end-to-end self-service synthetic data platform, which it calls the Data-as-Code solution, that generates realistic and high-variance vision data, allowing computer vision engineers to create high-fidelity synthetic data in a finely controlled and scalable manner.
What are the use cases for synthetic data?
Synthetic Data Use Case: In-Cabin Automotive
In-cabin automotive is a good example to better understand the role of Datagen. The term refers to what is happening inside the car, such as whether passengers are wearing their seat belts. Passengers and cars come in many forms, and that’s where artificial intelligence comes in handy. Based on some initial real-life 3D motion capture, Datagen allows its clients to generate the larger amounts of data they need to decide, for example, where airbags should be deployed.
Synthetic Data Use Case: Augmented Reality/Virtual Reality/Metaverse
Datagen is also dedicated to powering the metaverse with synthetic data. For virtual worlds to be truly immersive, this includes the ability to interact with virtual objects, optimize on-device rendering using accurate eye gaze estimates, realistic representation of user avatars, and create stable 3D digital overlays on top of the real world.
AI models are key to achieving these capabilities. But acquiring visual data to train these AI models is extremely challenging. It requires large amounts of facial and full-body data that can be accurately annotated in 3D for tasks such as hand pose and mesh estimation, full-body pose estimation, gaze analysis, SLAM, 3D environment reconstruction, and codec avatar creation.
Manual collection and annotation of such data is typically slow, costly, non-scalable, prone to privacy issues, and lacking in critical 3D annotations. Datagen data includes accurate representations of dynamic movements such as grips, gestures and eye movements. Customer development teams can use the platform to generate facial and full-body simulation data to quickly iterate on their models and improve their performance.
The next issue continues with other directions in AI investment.